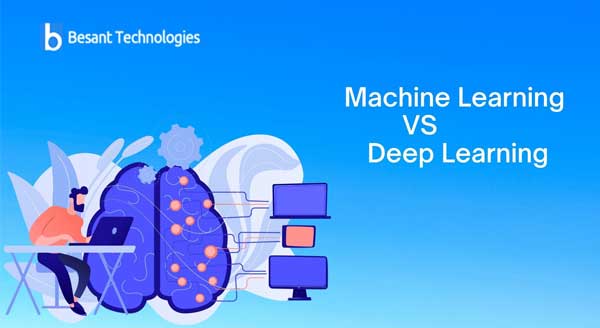
Machine Learning VS Deep Learning
In the world of AI, it has been seen that people use the terms machine learning and deep machine learning often interchangeably. But that’s not the right thing to do!
If you want to understand artificial intelligence better, you will have to be able to differentiate between these two vital ways in which computer systems learn. Thankfully, the concepts of machine learning and deep learning are not as complex as they might seem.
Put simply, machine learning is a subset of artificial intelligence. Whereas, deep learning is a subset of machine learning. So, both the concepts are interconnected in a way, but they are for sure not interchangeable. Now, let’s take a look at the most prominent factors on the basis of which they are distinguished from each other.
Speed of learning
Machine learning algorithms don’t take long to train because of fewer parameters. But the reverse is the case with deep learning. Prediction is also swifter in case of machine learning as compared to deep learning.
Dependency on hardware
To train machine learning models, you don’t need much. For instance, in order to train a classical model, one or more CPUs should suffice. However, you need hardware accelerators like TPUs or GPUs for training deep learning models. Not using such accelerators could cause deep learning models to take months to train.
Dependency on data
Deep learning algorithms are poor performers when the scale of data is small. Why? Because they need a massive amount of data to understand and identify patterns. Traditional machine learning algorithms are a far better performer in this scenario.
The factor of feature engineering
Feature engineering is a way of creating feature extractors by using domain knowledge. These feature extractors make data less complex and patterns more distinct to learning algorithms so that they can work better. But this process needs time and expertise.
In case of machine learning, an expert has to identify the major part of the applied features. These features are then hand coded according to the data and domain type. It is on the accuracy of the identified and extracted features that the performance of a machine learning algorithm depends.
Deep learning algorithms are ahead of traditional machine learning in that they themselves try to learn features from the available data. In deep learning, it’s not needed to develop new feature extractors for each and every problem.
Finding solutions to problems
When you solve a problem with a machine learning algorithm, you need to first break it up into different parts. These individual problem parts need to be solved separately. The solutions to these problems are then combined together to arrive at the final result. On the other hand, problem solving in case of deep learning is an end-to-end process.
Reasoning behind results/ interpretability
Interpretability is better in machine learning than in deep learning. For instance, when you use a deep learning algorithm to solve a problem, you are likely to get outstanding, near-human results. But you can’t easily interpret why it gave that result. After all, it was a complex neural network at play, and it’s hard to guess what the neurons involved were collectively doing to arrive at that result. But the traditional machine learning problem solving process is different. Algorithms like decision trees and logistic regression are generally involved which are guided by clear-cut rules. By studying them, one can easily tell why a particular result was obtained.
Machine learning and deep learning applications
Some of the most common areas where deep learning is used are speech recognition, natural language processing and image classification. Machine learning, on the other hand, is used in medical diagnosis, product recommendation, social media features, statistical arbitrage, and certain other classifications and predictions.
Final words
Both machine learning and deep learning have made it possible for machines to work almost like humans, making life hundred-fold easier. Some applications of this science can almost make your head spin. Take for instance the self- driving cars. They were probably beyond human imagination a few years back, and today they are a reality and are zipping away on the roads, fascinating onlookers. They are nothing but a result of deep learning. So, it’s only obvious that the future holds much more than what we can even imagine now. Hence, if you think this would be an interesting field to pursue a course in, then contact Besant Technologies right away. We, at Besant, can help you Fulfil your dreams of becoming an AI expert.